In the art and science of geodemography, the distance that potential customers travel to patronize stores or bank branches plays a key role in creating trade areas. Trade areas can be defined in many ways, with the simplest being a circle circumscribed around a store location. In practice, a perfectly circular trade area is rare; most are polygons that take into account distinctive demographics, man-made barriers and non-residential areas.
The main rationale for defining trade areas is to capture customer demographics. Regardless of shape, retail trade areas are generally created as tight areas that reflect reasonable travel distances for the store’s customers. By defining a trade area, marketers can gain an understanding of the demographics of the area’s residents, who are considered as representing the customers of the store.
Of course, if you already have comprehensive data on a store’s clients, you do not need to create a trade area to represent them—unless you are searching for new store locations.
The most common practice is to create a geodemographic profile that defines all the people or households in the trade area. Although this approach makes the assumption that all people in a trade area are equally likely to be patrons of the store—which is unlikely—it simplifies things greatly.
Simple trade areas of consumers closest to a store are an approximation because there are typically lots of non-customers inside the trade area and lots of actual customers outside of it. So how accurate—and thereby powerful—for applied use are the demographics in these trade areas?
Let us assume that, in a particular trade area, there are no alternative sister stores or true competitors to attract potential customers. (We’ll save the complicated issue of competition and cannibalization for another day.) Some retail analysts have defined “tapered demographics” for trade areas. In this approach, you take a conventional trade area and, rather than computing the demographics for the whole trade area by treating everyone equally, you compute a weighted demographic profile, giving more weight to those close to the store. The idea is that those who are closest to a store are more likely to patronize it, and therefore their demographics should be given more weight when creating a profile of a store’s customers.
But is this wise? Think about it. Isn’t it possible that the folks living close to the store patronize it not because the store is particularly attractive to their demographics but simply because it’s convenient?
A case can be made to give more weight to the demographics of those regular customers who live further away because they have a greater commitment to the store, as demonstrated by their willingness to incur greater costs in time and travel expense to patronize it.
Economic theory clearly supports this proposition. And the commitment of those customers often results from the store being more aligned with their demographics. These consumers are not as interested in convenience as they are in the specific products and services offered by the store.
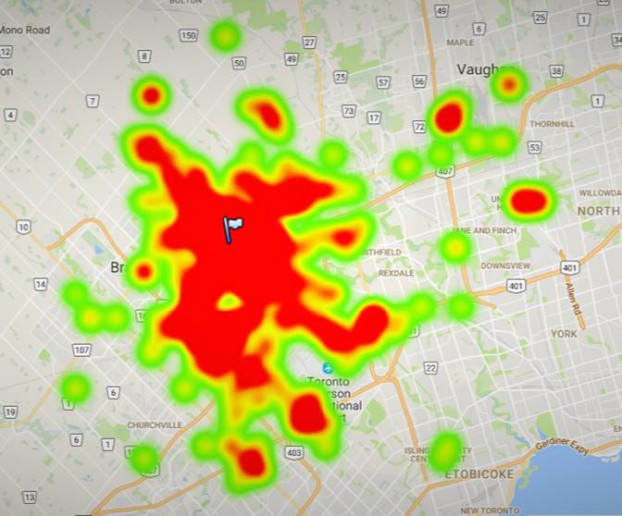
Density of all trip origins for one year to a retail site at the intersection of Airport Road and Queen Street East, Brampton.
Of course, there may be some very good reasons why some people travel further to patronize seemingly inconvenient stores: maybe their commute to work takes them near an otherwise inconvenient location, or perhaps they regularly visit an elderly aunt who lives near the store and they stop in to pick up last-minute gifts for her. On average, however, weighting those customers who live further out rather than closer in has some merit.
Testing the hypothesis that the greater-distance folks are more important than closer-in people typically requires a customer database with addresses, and you include the amount spent at the store in your data.
Other approaches can be more complex by taking into account elements such as recency, frequency and dwell time. Do the customers who travel further spend more money over an extended period of time than those who make shorter trips? Typically, the answer is yes. Taking this into account when developing the best geodemographic profiles means weighting distant consumers more.
If older folks travel farther than younger people who live closer, retail analysts should choose new store locations and create direct marketing that targets older customers.
Of course, close-in consumers, whatever their demographics, will always be critical to the performance of a store. But the argument for focusing on the demographics of distant consumers suggests that modifying conventional practice may be worthwhile.
—————————————-
Anthony Lea, Ph.D., is the Senior Vice President and Chief Methodologist at Environics Analytics.